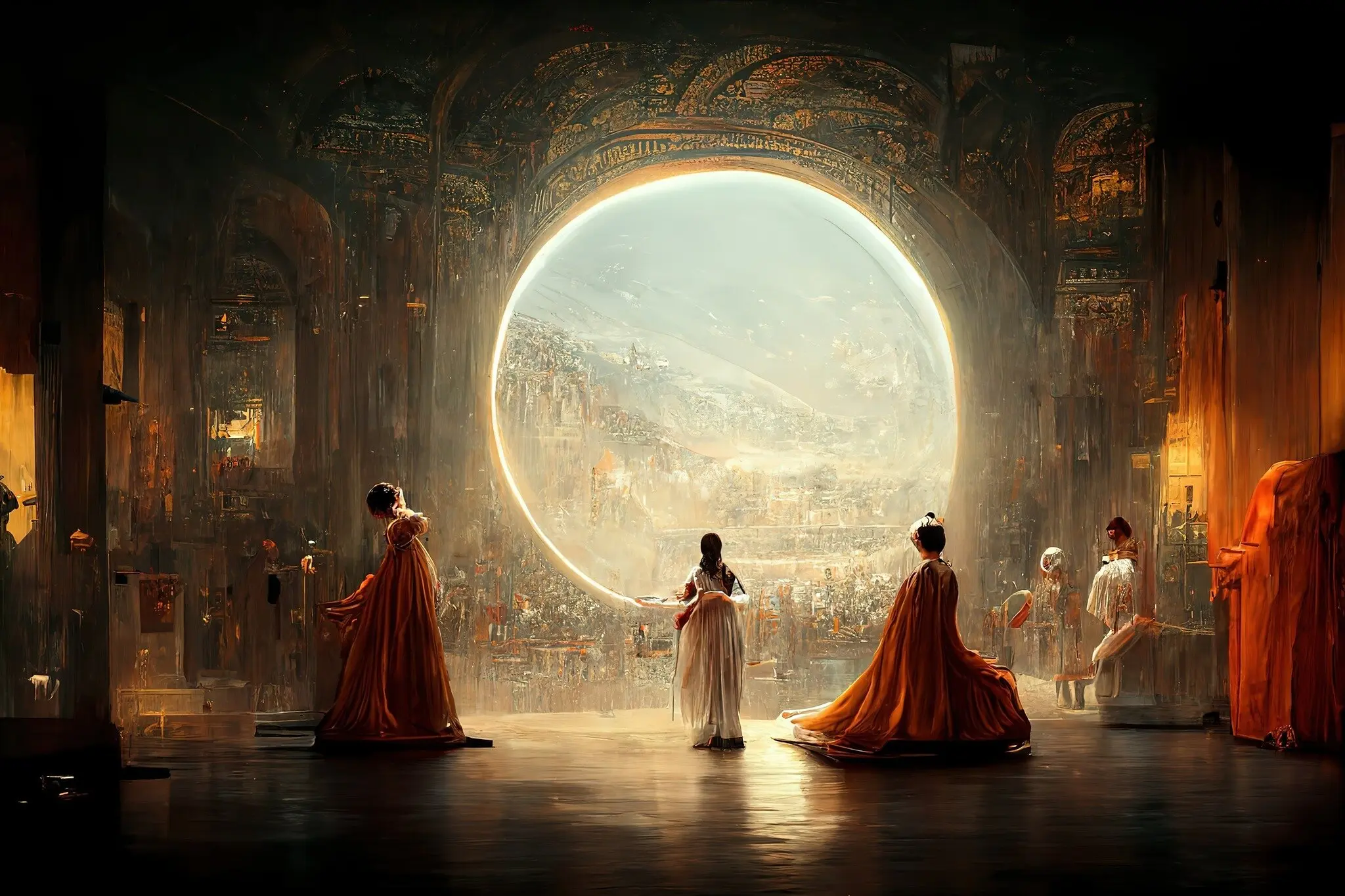
This artwork, "Théâtre D’opéra Spatial," wasn't just any piece at the Colorado State Fair.
Created by Jason Allen with the help of an AI art generator tool called Midjourney, it snagged a top award.
This is a big deal because it's not every day that an AI-made artwork wins something like this.
Some artists who make art the traditional way weren't too happy about it. They probably didn't expect an AI to come in and win a prize in an art contest.
But here's the interesting part: this shows that AI is really changing how we think about art.
It's not just about drawing and painting anymore. Now, computers and AI are joining in, and they're making stuff we never thought possible.
It's a whole new world for art with AI in it, blending traditional creativity with digital innovation.
What's more, it's not just about creating unique pieces of art; it's also a gateway for artists and creators to make money with AI art.
So, in this article, let's unpack this phenomenon. We're going to dive into AI art.
What is AI art? How does it work? And why is it getting so much attention?
What is AI Art?
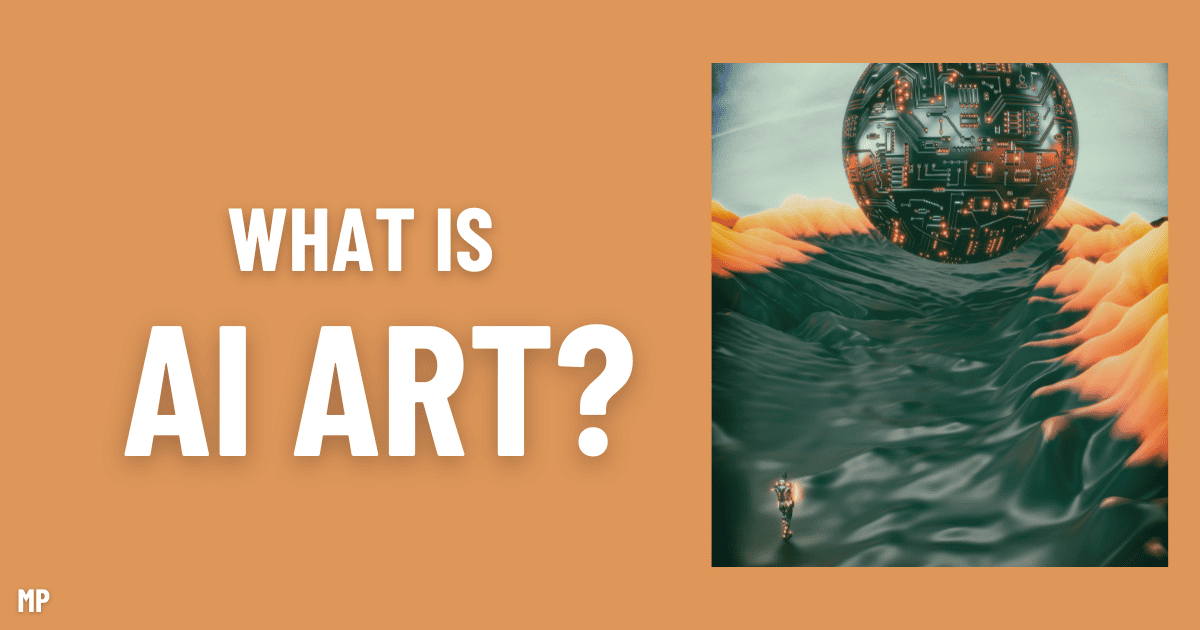
AI art is a technology where machines create images from text descriptions. It started in 2015 with AI transforming image labels into descriptions.
The idea evolved: why not generate images from text?
Early experiments were simple, like making a green school bus, something unusual.
These efforts set the stage for more complex creations, like "elephants flying in the sky" or "a vintage cat photo."
Nowadays, this technology has advanced significantly.
AI models like DALL-E 3 can create a wide variety of images based on text prompts.
They're trained on huge datasets, learning various styles and concepts.
A key part of this tech is "prompt engineering" – using the right words to guide the AI to produce specific images.
The images created are not just copies but new, original creations.
AI is a new way of combining human creativity with machine intelligence, pushing the boundaries of imagination.
How Does AI Art Work? The Technology Behind Artificial Intelligence Art
1. Data Collection
To create art, the AI needs to be trained with a large dataset.
This dataset consists of millions of existing art collected online, each paired with text descriptions.
These descriptions might be simple tags, detailed captions, or alt text used for accessibility. The AI uses this vast collection of image-text pairs to learn about the visual world.
2. Deep Learning
In the deep learning process, the AI tries to understand the relationship between images and their descriptions.
The AI starts by making many guesses about what different words mean in pictures. It's like playing a matching game, figuring out which words go with which images.
When it gets something wrong, it learns from that mistake. It keeps practicing, getting better and better, just like when you learn to do something new. Over time, the AI gets really good at matching the right words to the right pictures.
3. Feature Extraction
While training, the AI learns to pick out different details from pictures. These details can be basic things like colors and lines, or more complicated like textures and designs.
The AI gets better at noticing these details by looking at how the tiny dots of color (pixels) in the pictures are arranged.
For instance, it learns that pictures with a lot of yellow dots and long shapes are usually called bananas.
4. Latent Space
After the AI learns to recognize different details in images, it arranges them in a special latent space.
Think of this space as an imaginary map where similar details and ideas are placed close to each other. Every point in this space can be a picture the AI might create.
The way this space is set up is hard to explain in simple terms; it's made of complex math that the AI uses to tell different types of images apart.
5. The Diffusion Process
When the AI gets a text instruction (prompt) to make a new picture, it doesn't just find a matching picture it has seen before.
Instead, it goes to a spot in its special map (latent space) that fits the instruction. Then, it starts making the picture using a method often called diffusion.
This method begins with a random mess of dots (pixels) and slowly turns it into a clear picture.
The AI does this by slightly changing the random dots, pushing them to look more like the details and features it learned about earlier.
After many small changes, these adjustments result in a picture that matches the prompts.
6. Uniqueness and Variation
Because the diffusion process involves randomness, the AI will never create the exact same image twice, even with the same prompt.
Plus, different AI models, trained on different datasets or designed by different engineers, will have their own unique latent spaces. This means they will generate different images in response to the same prompt.
What types of AI are used to generate art?
AI used to generate art typically falls into several categories based on the techniques and algorithms they employ.
Here are some of the most common types:
Generative Adversarial Networks (GANs)
These are perhaps the most famous AI models for art generation.
GANs consist of two neural networks:
- The generator
- The discriminator
...which are trained simultaneously.
The generator creates images, while the discriminator evaluates them.
Over time, the generator improves at producing images that look like the training data, which can be anything from classical paintings to modern digital art.
Variational Autoencoders (VAEs)
VAEs are another generative model that can create new images by learning to encode data into a latent space and then decode from that space back into the original data space.
They are often used for creating variations of input images or generating new images that share characteristics with a training set.
Convolutional Neural Networks (CNNs)
While CNNs are primarily used for image recognition tasks, they can also be repurposed for art generation, especially when combined with techniques like style transfer, where one image's style is applied to another's content.
Recurrent Neural Networks (RNNs)
RNNs and their variants, like Long Short-Term Memory Networks (LSTMs), are indeed more commonly associated with tasks that involve sequences, like text generation, because they are designed to handle sequential data with dependencies over time.
However, their versatility allows them to be applied to any sequential data, including sequential art forms like animations, where the order of images is key.
They can also be used to create artworks that have a narrative or temporal dimension, such as comic strips or storyboards.
Transformers
Known for their performance in natural language processing, transformers can also be used for image generation.
They can handle data sequences, whether text for language models or pixels for images, making them versatile for various generative art tasks.
Neural Style Transfer
This technique uses neural networks to apply one image's artistic style to another's content.
It's not a generative model on its own but is often used in conjunction with other models to create stylized art.
Evolutionary Algorithms
These algorithms simulate the process of natural selection to generate art.
They start with a population of random images and iteratively select, mutate, and recombine them to create aesthetically pleasing images or meet specific criteria.
DeepDream
Originally developed by Google, DeepDream modifies images to induce dream-like hallucinogenic appearances in the style of the training images.
It's known for creating surreal and psychedelic imagery.
Creative Adversarial Networks (CANs)
A variation of GANs, CANs are designed to produce art by not only learning from a dataset but also seeking to deviate from the styles of the dataset, thus introducing a level of creativity and novelty.
These artificial intelligence models can be trained on various datasets and fine-tuned to produce specific styles or types of art.
The resulting artworks can range from paintings and drawings to digital and even interactive art.
The Bittensor network can leverage the distributed computing power of miners to train and run these complex models, pooling together resources to generate high-quality art efficiently.
Examples of AI Art
1. Edmond de Belamy
This portrait, created by the Paris-based art collective Obvious, was generated using a GAN and sold at Christie's auction for over $400,000.
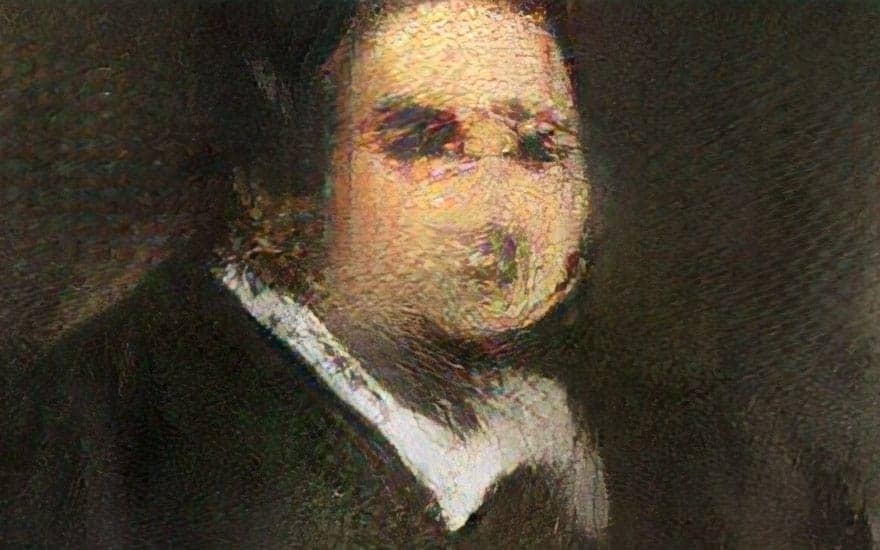
2. The Next Rembrandt
A project that used machine learning algorithms to analyze Rembrandt's paintings and create a new, original artwork in the style of the Dutch master.
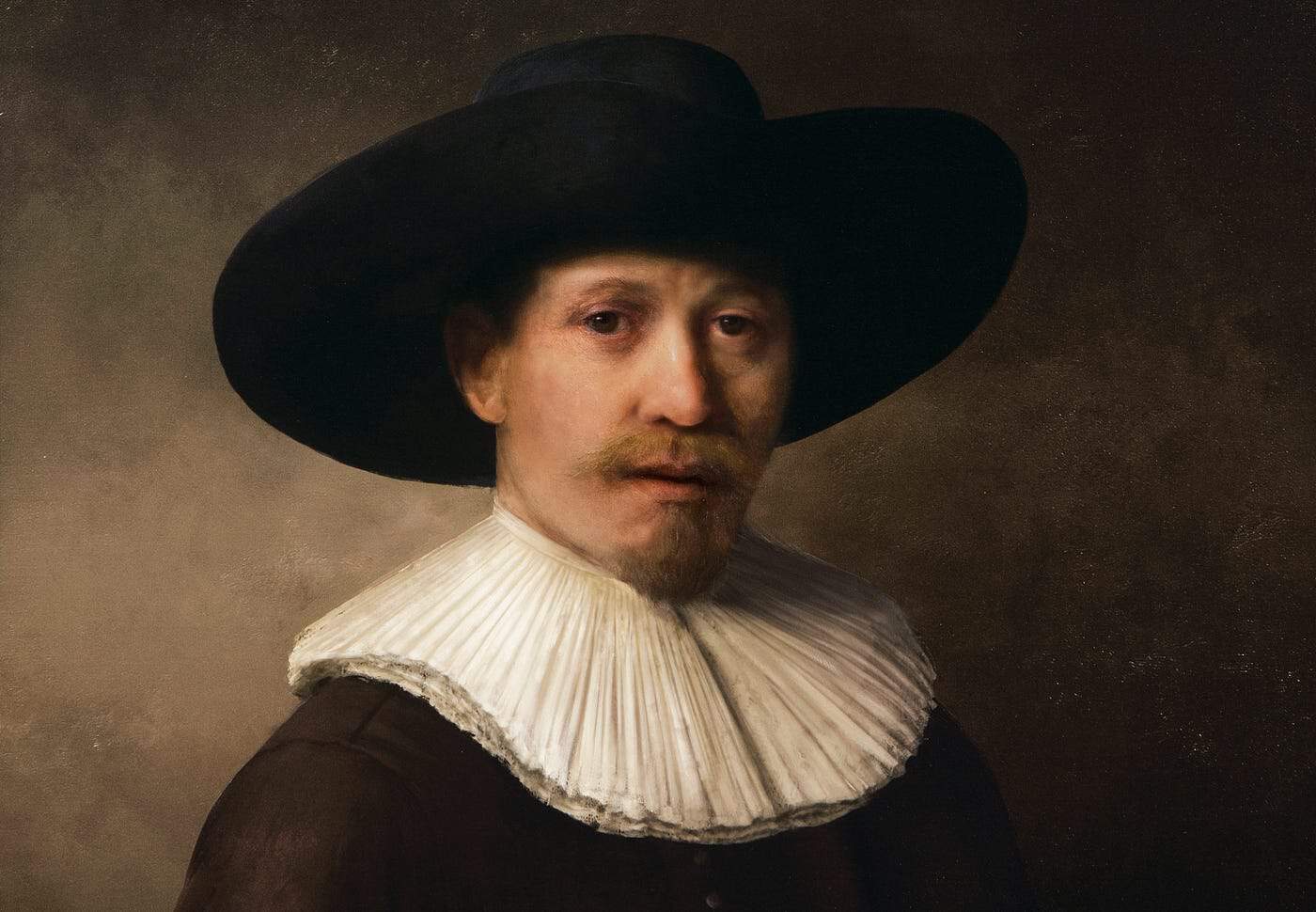
How are Human Artists Using AI?
Human artists use AI to:
- Collaborate: They team up with AI to create new pieces, with AI suggesting ideas and artists adding their touch.
- Generate Patterns: Artists use AI to make complex designs and shapes quickly.
- Create Interactive Art: They build installations where AI responds to viewers, changing the art in real time.
- Visualize Data: AI helps artists turn big data into visual stories or patterns.
- Experiment: Artists explore new styles and visuals that AI can generate, which are beyond traditional human concepts.
- Personalize: AI tailors art to react to individual viewers' tastes or emotions.
In short, artists are using AI as a tool to expand their creative possibilities, make interactive and personalized art, and explore new forms of expression.
Final Thoughts
AI artwork is shaking things up, showing us that art isn't just about paint and canvas anymore.
With AI tools, anyone can dive into art-making, explore new styles, and even stumble upon happy accidents that a human hand might not make.
It's a game-changer, making art more interactive and personal. As we move forward, AI is going to keep making waves, and it's exciting to think about what's next.
Whether you love it or you're skeptical, one thing's for sure: AI art is opening doors we didn't even know were there.